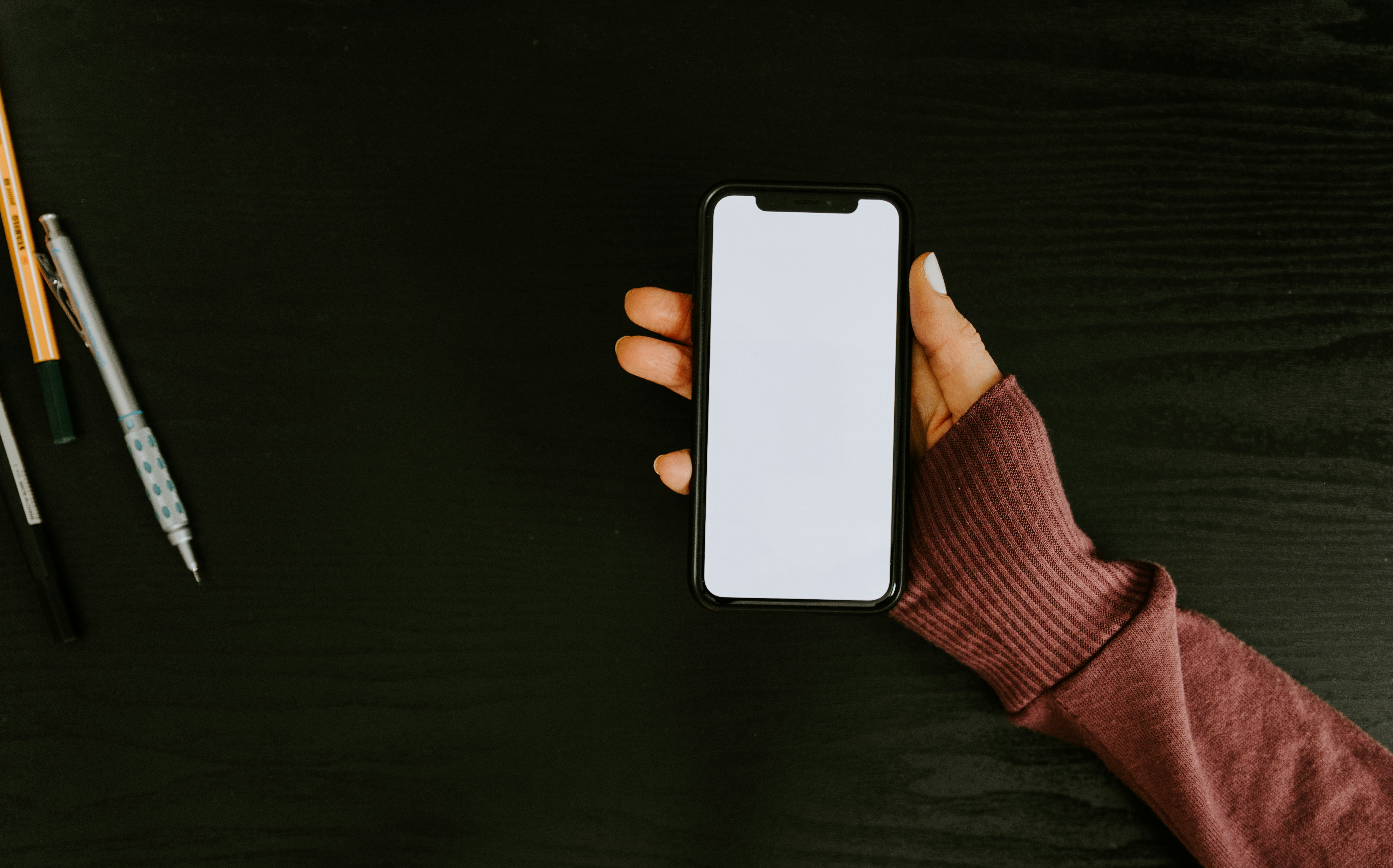
In an era where virtual classrooms, online assessments, and digital portfolios have become increasingly common, educators now have unprecedented access to massive amounts of student data. This surge in information is revolutionizing the way schools and learning platforms operate. By applying data science principles to this wealth of data, educators can craft more personalized lessons, develop adaptive testing tools, and refine teaching strategies to better meet learners’ individual needs.
At the heart of this movement is Ben, a dedicated data scientist and mentor at Nova Scholar Education. Drawing on his extensive background in mathematics, educational technology, and advanced data analytics, Ben guides students through the nuances of data science. From mastering fundamental programming techniques to interpreting complex datasets, his mentorship helps future innovators acquire the critical thinking and analytical skills necessary for today’s increasingly data-centric world.
Yet Ben’s work represents more than just crunching numbers—he champions an approach that merges ethical considerations, robust project management, and a genuine passion for discovery. Students under his tutelage don’t just learn to program; they learn to ask meaningful questions, design responsible research methods, and draw data-driven conclusions that can shape the future of education.
Ben’s Journey: From Teaching to Data Science
The Early Years: Teaching and Curriculum Innovation
Ben’s path to becoming a data science mentor began in the traditional classroom. Through Teach for America, he taught high school mathematics to underserved communities, quickly realizing how a rigid curriculum and a one-size-fits-all approach left many students unengaged or behind. Motivated by the desire to make education more equitable and personalized, he looked for ways to use data to illuminate student struggles and strengths.
Later, he joined Khan Academy, where he tackled the challenge of producing data-driven learning resources. At Khan Academy, Ben delved into detailed student performance metrics, investigating how learners interacted with online lesson modules, practice problems, and instructional videos. This experience granted him a front-row seat to the power of real-time analytics in identifying educational gaps, refining difficulty levels, and ultimately improving academic outcomes across diverse populations.
“I realized early on that conventional tests and homework scores only told a fraction of each student’s story,” Ben recalls. “By analyzing how students arrived at answers or where they repeatedly got stuck, I could uncover hidden misconceptions and adapt lessons accordingly.”
This realization fueled his growing passion for bridging educational theory with data analytics. Witnessing firsthand how data-based insights could transform a student’s trajectory solidified his commitment to expanding data science in schools.
Advancing Technical Expertise: Harvard Data Science Fellowship
Driven to deepen his understanding of advanced analytics, Ben pursued a two-year Data Science Fellowship at Harvard University. Immersing himself in cutting-edge research, he worked with extensive educational datasets—from student reading comprehension logs to longitudinal analyses of math interventions. By applying machine learning models, he uncovered patterns tied to student success, including the optimal frequency of practice problems and the types of feedback that accelerate mastery.
“We have an unprecedented amount of educational data at our fingertips,” Ben explains. “My focus is on leveraging this data to refine instructional design and assessment methods, ensuring that every lesson is truly tailored to the learner’s progression.”
At Harvard, Ben collaborated with educators, data scientists, and product designers to develop prototypes of adaptive learning tools. His research not only benefited academic circles but also caught the attention of educational startups eager to incorporate algorithmic assessments into their platforms. This cross-pollination of ideas laid the foundation for his future role at Nova Scholar Education.
How Data Science Is Transforming Education
Personalized Learning Through Adaptive Technologies
One of the most promising impacts of data science on education is the rise of adaptive learning systems. These technology-driven platforms track a student’s every interaction with learning materials—such as response times, accuracy, and even the order in which questions are tackled. By analyzing these variables in real time, the software can dynamically adjust lesson content. For instance, if a learner shows mastery over basic algebraic concepts, the program will immediately introduce more complex equations. Conversely, if the system detects repeated errors in geometry, it may provide additional instructional resources or slower-paced review.
Such precision-driven instruction reduces frustration for advanced learners who might otherwise feel bored and unchallenged, while giving struggling students extra time to grasp fundamental concepts. Ultimately, adaptive platforms serve as a complement to traditional instruction, allowing teachers to focus on higher-level thinking activities.
Enhancing Assessment and Concept Mastery
Traditional tests often offer a binary snapshot of what a student does or does not understand. Data science, however, enables educators to dig deeper. By evaluating a student’s step-by-step process—how they tackled each question, the time spent on certain problem types, or the hints they requested—educators gain richer insights into a learner’s thought patterns.
Ben has brought these techniques to his mentorship at Nova Scholar. Under his guidance, students learn to analyze real-world assessment data, pinpointing trends such as frequent student errors, the complexity level of certain question types, or how time of day impacts performance. These findings enable more targeted revisions of teaching materials, ensuring that tricky concepts receive extra emphasis and that each exam question truly measures critical skills rather than rote memorization.
Data-Driven Curriculum Development
Beyond individual assessments, data science influences broader curriculum design. By studying which topics students find consistently challenging, curriculum developers can reorder units to build on foundational concepts more effectively. They might integrate multimedia resources—videos, interactive simulations, or peer collaboration exercises—precisely where students need extra help. This cyclical feedback loop ensures that teaching materials evolve continually, staying aligned with learners’ emerging needs and the realities of an ever-changing world.
Mentorship at Nova Scholar Education: Nurturing the Next Generation of Data Scientists
What Makes Nova Scholar Unique?
Nova Scholar Education distinguishes itself through a project-based mentorship approach that offers students individualized attention and a deep dive into advanced topics. Importantly, mentors hail exclusively from Stanford, Harvard, Yale, Princeton, and MIT, ensuring that learners receive top-tier guidance grounded in cutting-edge research and industry expertise.
Programs include:
- Nova Fundamentals (1 Month): Tailored for middle school students, this short-term program introduces foundational research and problem-solving techniques, often incorporating entry-level data science concepts.
- Nova Research (2.5–5 Months): Catering to middle and high school students, Nova Research fosters independent, in-depth exploration of STEM and humanities topics, blending critical thinking with tangible skills like data analysis.
- Nova Patent (2–4 Months): Geared towards high school students interested in innovation, this program covers patentable projects and advanced research methodologies, equipping participants with the know-how to transform ideas into intellectual property.
Within these structured programs, students are encouraged to stretch beyond classroom norms—exploring specialized datasets, building predictive models, or even designing apps related to their research fields. Ben’s role involves both guiding project direction and ensuring students integrate ethical practices, robust methodologies, and effective communication strategies.
Ben’s Approach to Mentorship
At Nova Scholar, Ben sees mentorship as a balance between structured frameworks and individual autonomy. He provides scaffolding in areas like statistical modeling, data cleaning, and machine learning, but gives students the freedom to drive their own investigations. According to Ben:
“Data science thrives on hands-on experimentation. The real learning happens when students wrestle with unexpected results, debug their algorithms, and pivot their research questions based on new insights.”
In practice, this can look like:
- Regular Check-Ins: Ben and his mentees review progress weekly, setting milestones and resolving blockers.
- Peer Collaboration: Students are encouraged to share findings with each other, replicating the collaborative environment of professional data science teams.
- Reflective Learning: Journaling or blogging about challenges helps learners articulate their thought processes and measure personal growth over time.
Real-World Projects: Bringing Data Science to Life
Ben’s students often tackle a range of fascinating topics, reflecting the interdisciplinary essence of data science. Here are a few illustrative projects:
Sports Analytics
- Predicting Football Play Calls: By reviewing thousands of past football games, one group used machine learning algorithms to anticipate whether a team would opt for a pass or run in specific game scenarios. Through careful feature engineering—incorporating factors like down, yardage, field position, and even weather—students refined their model’s accuracy and offered strategic insights that could influence real-time coaching decisions.
- Analyzing Pitching Trends in Baseball: Another cohort focused on a massive historical dataset of pitches from professional leagues. They discovered patterns linked to pitch velocity, spin rate, and batter tendencies, ultimately shedding light on the evolution of pitching strategies over decades.
Personality Psychology
- Big Five Personality Traits: Ben supervised students investigating over a million anonymized survey responses tied to the Big Five traits: openness, conscientiousness, extraversion, agreeableness, and neuroticism. By correlating these traits with self-reported habits—like exercise frequency or social media usage—they unearthed intriguing relationships. The project underscored the complexity of psychological data, challenging students to account for biases and outliers in large-scale datasets.
- Behavioral Predictions: Some students ventured into designing rudimentary predictive models to forecast how personality traits might align with real-world outcomes such as college major selection or career satisfaction. While these projects were exploratory, they offered a glimpse into how big data can illuminate human behavior.
Educational Data Science
- Improving Assessment Design: Some of Ben’s mentees analyzed extensive logs from digital math assessments. They probed how question phrasing, hint frequency, and the order of problems affected student performance. This analysis contributed to actionable recommendations for reordering or rewording tricky items in the test bank.
- Adaptive Tutorial Systems: Students experimented with creating mini-adaptive platforms, identifying when learners were ready to advance or needed remedial content. This project required collaboration with software developers, reflecting the real-world teamwork needed to bring educational tech products to life.
The Lifecycle of a Data Science Project at Nova Scholar
Students at Nova Scholar follow a rigorous project workflow that mirrors professional practices in tech companies, academic labs, and research institutions:
- Identify a Research Question
Learners brainstorm topics that inspire them—be it sports, psychology, or educational practices. They refine broad ideas into feasible, data-oriented questions. - Gather and Clean Data
Mentees acquire relevant datasets, grappling with raw files that often have missing entries, inconsistencies, or errors. They learn the critical skills of preprocessing, normalization, and documenting their steps for reproducibility. - Explore and Analyze Data
Using statistical tools (e.g., Python’s pandas or R’s tidyverse), they examine distributions, correlations, and outliers. Initial visualizations, such as histograms or scatter plots, guide them toward deeper questions. - Build and Test Models
In predictive projects, this stage involves training machine learning algorithms—random forests, gradient-boosted models, or neural networks—and carefully evaluating performance using metrics like accuracy, precision, or recall. - Communicate Findings
Students learn how to succinctly present results through data visualizations, written reports, and oral presentations. This step emphasizes clarity and context, ensuring that audiences grasp the significance of the discoveries. - Iterate and Refine
Rarely does a project unfold perfectly on the first try. Students revisit earlier steps, refining their question, cleaning methods, or model parameters in response to new insights.
Beyond the Code: The Hidden Curriculum of Data Science
Ben emphasizes that technical prowess is just one piece of the puzzle. Equally important are intangible qualities and practices that distinguish exceptional data scientists:
- Project Management
Students learn to set clear goals, create realistic timelines, and break large tasks into manageable segments. Effective planning helps them avoid scope creep and meet deadlines—key skills in any data-driven profession. - Ethical Awareness
Data can reveal powerful insights, but it also carries risks. Ben coaches learners to spot biases in datasets and to weigh the potential impacts of their findings on communities. They delve into real case studies where algorithms reinforced racial or gender stereotypes, discussing how to mitigate such pitfalls. - Collaboration and Version Control
In a professional environment, data science rarely happens in isolation. Students practice using platforms like GitHub to track code changes and facilitate teamwork, simulating industry-standard collaboration. - Iterative Problem-Solving
A hallmark of advanced data scientists is the willingness to experiment, fail fast, and learn from missteps. By viewing every error as a clue rather than a setback, students develop the resilience needed to navigate complex analyses.
The Impact of Personalized Mentorship on Student Success
Unlike large lecture halls or massive online courses, Nova Scholar’s mentorship model offers an individualized experience. Students receive regular feedback from experts who have a nuanced understanding of their progress, learning styles, and unique goals.
“The most transformative moments in my mentorship occur when students hit a snag,” Ben notes. “They must pause, rethink, and approach a problem from a fresh angle. That’s where true learning happens—where data analysis meets creativity.”
By taking on real-world problems, learners develop a sense of ownership that fuels deeper engagement. This autonomy and personalization often lead to:
- Higher Retention of Skills: Concepts in statistics or machine learning feel tangible when tied to personal interests. Students recall what they’ve learned because they’ve applied it to projects that genuinely matter to them.
- Confidence in Problem-Solving: Tackling complex data sets can be intimidating at first, but successfully navigating them builds self-assurance. Students realize they can tackle ambiguity—a critical skill in advanced academics and many 21st-century careers.
- Better College and Career Preparedness: Whether they pursue STEM degrees or venture into social sciences, students with data science experience stand out. Many have cited Nova Scholar projects in college applications, highlighting their capacity for independent research, critical thinking, and teamwork.
Actionable Insights: How to Incorporate Data Science into Your Learning Journey
Even if you’re not enrolled in a dedicated mentorship program, there are several ways to harness the power of data science for educational or personal growth:
- Start Small
Dive into publicly available datasets—such as those from Kaggle or government open data portals—and try basic analyses. Simple questions often lead to surprising discoveries. - Focus on Fundamentals
Build a strong foundation in core skills like statistics, algebra, and coding in Python or R. Understanding these basics will make advanced concepts like machine learning far more approachable. - Collaborate with Peers
Join local clubs, online communities, or academic meetups focused on analytics and data science. Sharing insights or debugging code with peers accelerates learning. - Explore Real-World Applications
Tie your projects to personal interests—sports, social issues, health, or even music trends. Connecting data analysis to genuine passions keeps motivation high. - Seek Mentorship
If possible, find a mentor—whether through school programs, community organizations, or virtual platforms—who can provide expert guidance and help you avoid common pitfalls.
The Future of Data-Driven Education
As big data, machine learning, and advanced analytics continue to reshape industries worldwide, the education sector stands on the cusp of its own data-driven revolution. Programs like Nova Scholar Education exemplify how mentorship, supported by rigorous project-based learning, can empower the next generation of scholars, innovators, and change-makers.
Ben’s journey from traditional teaching to advanced data science underscores the need for educators who understand both pedagogy and analytics. By collecting and interpreting data on how students learn, mentors can tailor curricula and assessments in ways that foster deeper engagement and mastery. Instead of relying on broad, one-size-fits-all approaches, data analytics paves the way for nuanced, individualized strategies that resonate with each learner.
Moreover, when students themselves become data storytellers—proficient in cleaning raw data, building predictive models, and presenting actionable findings—they’re not just building technical portfolios. They’re also cultivating critical thinking, ethical awareness, and problem-solving finesse that translate into lasting career and life advantages. In a digital age where numbers guide decisions across every industry, these skills are invaluable.
“Data science isn’t just about crunching numbers,” Ben concludes. “It’s about leveraging insights to improve lives and shape the future. At Nova Scholar, we want students to be the kind of problem-solvers who aren’t afraid of complexity and can envision new possibilities.”
By integrating adaptive learning technologies, informed curriculum design, and a robust mentorship framework, educational institutions can unlock the potential of data science in ways that benefit students everywhere. As this field continues to expand, learners who embrace data-driven methods today stand poised to become the innovators, researchers, and thought leaders of tomorrow.